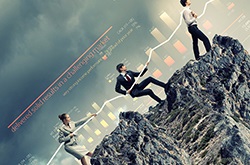
Here’s Why There is No Substitute for Good Data
Author: Aaron Smith
Increased proliferation of technology across devices and people has exposed previously unseen business models, new marketing strategies and markets, and experiment-based management strategies. All of this information inherently springs from millions of bits of data that arise as a result of connectivity.
When harnessed actionably, continually growing data sets can be used to enhance IoT, Artificial Intelligence, use of targeted marketing and digitization for organizations that are relatively new to these age-altering technologies. Since data volume drives AI and cognitive functions of Machine Learning systems, an investment in data is a direct investment into futuristic technology.
With several small and big companies making data use vital to their everyday operations, data management has become a competitive differentiator in the market. Since data sets provide essential insight into the working of even the bottom lines of organizations, they are no longer abstract or theoretical.
Practical use of data in markets helps in client acquisition, management, and marketing. Rather than alienating data from other functions of a brand, it is important to incorporate it into content creation, management, and client servicing. This creates the pressing need to adopt and manage data in the most efficient manner. Brands that successfully understand the relevance of a data set and implement results or insights gained from it into their operations see an increased level of market success.
However, the first step to effective data management is the acquisition of high-quality data. Companies must understand that not all data available to them is useful or relevant to their unique needs. Data that is high-quality, accurate and relevant is “good data” whereas every other data set is classified as bad.
Why Do You Need Good Data?
The completeness, relevancy, accuracy, and validity of a data set all contribute toward its quality.
An inaccurate piece of information is not only useless but also significantly damaging. “Garbage in – Garbage Out is a famous maxim of the software world equally valid for data management. If bad data goes to management teams, it takes a lot of redundant time and effort to be filtered. According to a report published by the Harvard Business Review, lousy-quality data delays or undermines the efficiency of Machine Learning in the business world.
If a data set is not good, it triggers a chain of bad performances within an organization. Data management teams receive incomprehensive and incompatible data which they then use to prepare incomplete or inaccurate reports. This faulty report later gets forwarded to customer servicing and product development and management teams who work on it only to arrive at inherently wrong solutions.
Over time, the negative effect such data sets have on the overall performance of the said organization only intensifies. This example is illustrated in a Forrester research – one-third of data analysts use 40% of their time to validate and verify data. The same data is later used for strategic decision-making, which often turns out to be potentially damaging. Therefore, bad data adversely affects both productivity and performance.
When data analysts and managers do not have to navigate through complex and inefficient data sets for hours every day, their confidence levels to produce valuable reports increase. Good data, therefore, allows for zero decision-making risks and a decrease in guesswork. Since good data is accurate and describes real-world conditions perfectly, wrong insights can never be derived from it. Moreover, the validity of such data sets can be tracked easily, and they can be verified several times during a single management cycle, preventing questionable decisions.
How Does Good Data Save Costs?
Good data directly impacts the financial workings of an organization. It reduces overspending or underpayments made as a result of lost time and effort, streamlines performance, decreases operating costs and penalties, and enhances market reputation.
Since revenue leakage and overhead costs of bad data are eliminated, companies that use good data sets see an immediate improvement in revenues. An IBM report further clarifies that small and big companies lose about $3.1 trillion every year due to bad data. This loss comes from a variety of factors, including wasted time and efforts sorting through bad data sets.
According to an Experian report, a bad data set impacts the bottom line of about 88% of organizations directly and brings about 12% of revenue loss. The quality of data scientists employed by an organization does not matter when erroneous decisions are made solely because of bad data, as was the case with Apple. When the company rolled out its Maps feature in the last decade, its inefficiency became clear very shortly. Later reports revealed that Maps failed drastically because it was based mainly on bad data. Later efforts to improve it were also based on inaccurate data sets and the company eventually withdrew the application. This debacle made apple lose millions in revenue and even more in reputation.
Bad data acts like a clickbait. It appears alluring and cheap at first, with many data providers even offering flexible terms of service. However, the problem with it gets realized only when its use has significantly progressed. The initial savings on a bad data set are rendered moot as everyday revenue loss increases. Whereas revenue can even be recovered over time, lost reputation is harder to regain.
The future for all brands, regardless of their size, will entail the adoption of good-quality data as a competitive necessity. By 2025, data monetization will likely become the most significant revenue source for organizations. Carefully allocating funds to the acquisition and management of high-quality datasets is much better than saving on bad data that only causes later monetary damage.
Keeping the significance of good data and the adverse effects of bad data in mind, brands must realize that highly-efficient data management software or the best-trained data scientists cannot act as substitutes for good data.
For a secure and successful future, brands must start setting clear objectives for data acquisition and management. Classifying teams that segregate bad data from a good one is also a useful methodology for detecting faults in data sets early on. Centralized and certified data is the answer to all corporate success-related questions in the future. Brands looking to escape stagnancy must, therefore, direct their best resources to recognize, acquire and incorporate only good data.